2D probabilistic undersampling pattern optimization for MR image reconstruction |
| |
Affiliation: | 1. College of Information Science and Electronic Engineering, Zhejiang University, Hangzhou, China;2. Department of Physical Medicine and Rehabilitation of The Affiliated Sir Run Run Shaw Hospital And Interdisciplinary Institute of Neuroscience and Technology, Zhejiang University School of Medicine, Hangzhou, China;3. Key Laboratory of Biomedical Engineering of Education Ministry, College of Biomedical Engineering and Instrument Science, Zhejiang University, Hangzhou, China;4. Department of Neurosurgey, Provincial Hospital Affiliated to Shandong First Medical University, Jinan, China;5. School of Computer Science and Engineering, Beihang University, Beijing, China;1. University of Paris-Saclay, CentraleSupélec, CVN, Inria, 09 Rue Joliot Curie, Gif-sur-Yvette 91190, France;2. GE Healthcare, 283 Rue de la Minière, Buc 78530, France;1. Department of Computer Science, University of North Carolina, Chapel Hill, NC 27599, USA;2. Department of Biomedical Engineering, University of North Carolina, Chapel Hill, NC 27599, USA;3. Department of Biomedical Engineering, University of Virginia, Charlottesville, VA 22904, USA;4. Department of Radiology, University of North Carolina, Chapel Hill, NC 27599, USA;5. Biomedical Research Imaging Center (BRIC), University of North Carolina, Chapel Hill, NC 27599, USA;1. Electrical Engineering (EE) Department, Indian Institute of Technology (IIT) Bombay, Mumbai, India;2. Computer Science and Engineering (CSE) Department, Indian Institute of Technology (IIT) Bombay, Mumbai, India |
| |
Abstract: | 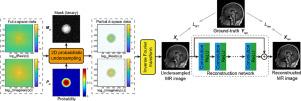 With 3D magnetic resonance imaging (MRI), a tradeoff exists between higher image quality and shorter scan time. One way to solve this problem is to reconstruct high-quality MRI images from undersampled k-space. There have been many recent studies exploring effective k-space undersampling patterns and designing MRI reconstruction methods from undersampled k-space, which are two necessary steps. Most studies separately considered these two steps, although in theory, their performance is dependent on each other. In this study, we propose a joint optimization model, trained end-to-end, to simultaneously optimize the undersampling pattern in the Fourier domain and the reconstruction model in the image domain. A 2D probabilistic undersampling layer was designed to optimize the undersampling pattern and probability distribution in a differentiable manner. A 2D inverse Fourier transform layer was implemented to connect the Fourier domain and the image domain during the forward and back propagation. Finally, we discovered an optimized relationship between the probability distribution of the undersampling pattern and its corresponding sampling rate. Further testing was performed using 3D T1-weighted MR images of the brain from the MICCAI 2013 Grand Challenge on Multi-Atlas Labeling dataset and locally acquired brain 3D T1-weighted MR images of healthy volunteers and contrast-enhanced 3D T1-weighted MR images of high-grade glioma patients. The results showed that the recovered MR images using our 2D probabilistic undersampling pattern (with or without the reconstruction network) significantly outperformed those using the existing start-of-the-art undersampling strategies for both qualitative and quantitative comparison, suggesting the advantages and some extent of the generalization of our proposed method. |
| |
Keywords: | |
本文献已被 ScienceDirect 等数据库收录! |
|